How Binomial Distribution Revolutionizes Finance, Investment, and Risk Management
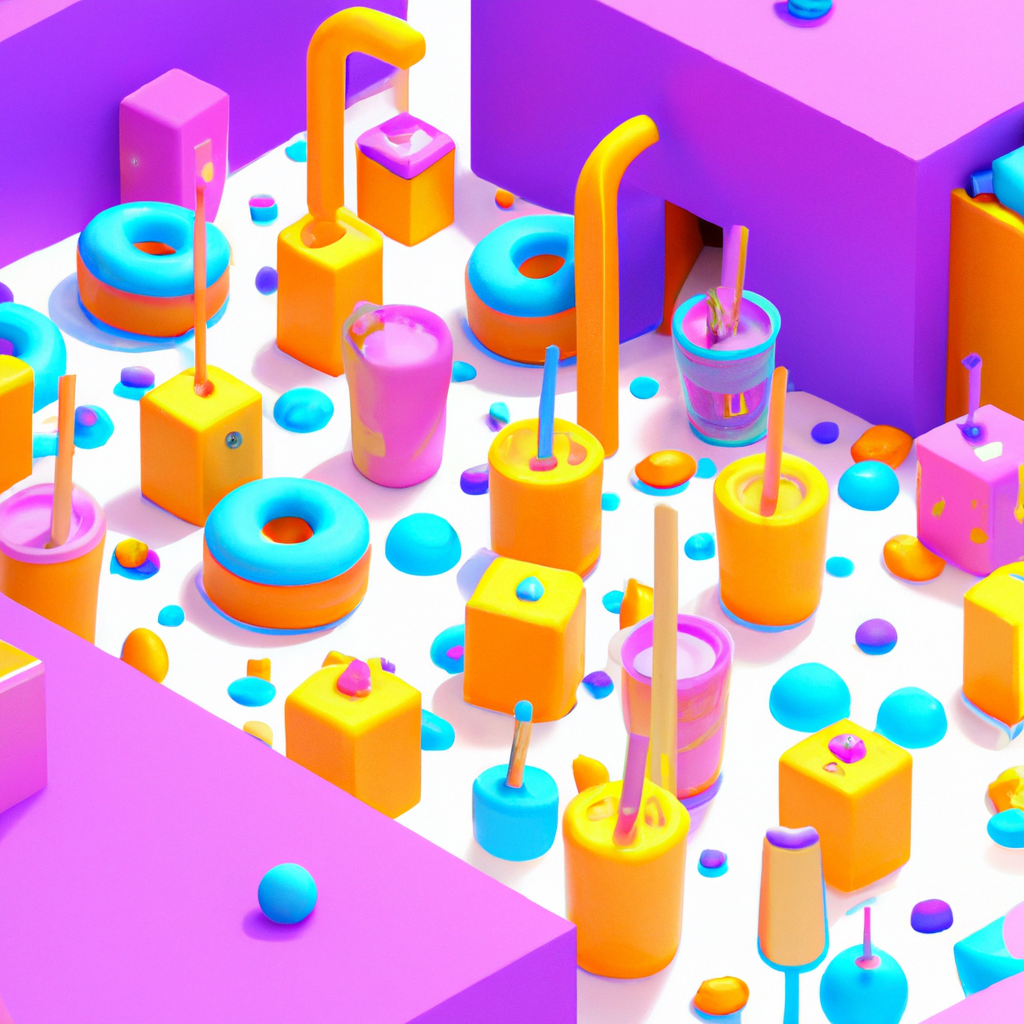
Understanding Binomial Distribution
Definition and Formula
The binomial distribution is a discrete probability distribution that models the number of successes in a sequence of ( n ) independent trials, where each trial has a constant probability ( p ) of success. The key parameters are:
- Understanding Appraisal Costs: How Quality Control Expenses Impact Your Business Bottom Line
- Understanding the Boom and Bust Cycle: Navigating Financial Market Fluctuations
- Mastering Backward Integration: How Companies Gain Control and Boost Efficiency in Their Supply Chains
- What is NFT Marketplace? – ZCrypto
- Mastering Activity-Based Budgeting (ABB): A Comprehensive Guide to Cost Optimization and Strategic Financial Planning
-
Number of trials (( n ))
Bạn đang xem: How Binomial Distribution Revolutionizes Finance, Investment, and Risk Management
-
Probability of success (( p ))
-
Number of successes (( k ))
The binomial probability formula is given by:
[ P(X = k) = \binom{n}{k} p^k (1-p)^{n-k} ]
where ( \binom{n}{k} ) is the binomial coefficient, representing the number of ways to choose ( k ) successes out of ( n ) trials.
Key Characteristics
One of the fundamental characteristics of binomial distribution is the independence of trials. Each trial is independent of the others, meaning that the outcome of one trial does not affect the outcome of another. Additionally, the probability of success remains constant for each trial.
The expected value (( E(X) )) and variance (( Var(X) )) of a binomial distribution are given by:
[ E(X) = np ]
[ Var(X) = np(1-p) ]
These metrics provide insights into the central tendency and dispersion of the distribution.
Applications in Finance
Risk Assessment in Lending
Banks use binomial distribution to estimate the likelihood of borrower default. By modeling the probability of default as a series of independent trials, banks can calculate the expected number of defaults and manage lending risks more effectively. For example, if a bank has a portfolio of 100 loans with a 5% probability of default for each loan, the binomial distribution can help predict the expected number of defaults and guide lending decisions.
Credit Scoring Models
Credit scoring models often employ binomial distribution to predict creditworthiness. These models analyze various factors such as payment history, credit utilization, and other financial metrics to assign a credit score. The binomial distribution helps in predicting the likelihood of a borrower being classified as high-risk or low-risk based on these factors.
Options Pricing and Hedging
In options pricing, the binomial options pricing model is widely used. This model assumes that the price of the underlying asset follows a binomial distribution over discrete time periods. By calculating the probabilities of different price movements, investors can determine the fair value of options and develop hedging strategies to manage risk.
Fraud Detection in Banking
Binomial distribution aids in fraud detection systems by distinguishing between legitimate and fraudulent transactions. By setting detection thresholds based on binomial probabilities, banks can balance the risk of false positives (legitimate transactions flagged as fraudulent) and false negatives (fraudulent transactions missed).
Applications in Investment
Sales Forecasting and Inventory Management
In sales forecasting, binomial distribution can be used to predict demand and manage inventory effectively. For instance, a retailer might use binomial distribution to estimate the probability of selling a certain number of units within a given period. This helps in making informed investment decisions regarding inventory levels.
Market Research and Surveys
Market research often involves analyzing binary outcomes such as customer preferences or purchase intentions. Binomial distribution helps in interpreting these data to inform investment strategies. For example, if a survey indicates that 60% of customers prefer a new product feature, the binomial distribution can help estimate the confidence interval around this percentage.
Employee Turnover Analysis
Xem thêm : How to Calculate Break-Even Price: A Comprehensive Guide for Business and Investment Success
Predicting employee turnover rates is crucial for human resource management and workforce development investments. By applying binomial distribution to historical data on employee turnover, companies can estimate future turnover rates and their financial implications.
Applications in Risk Management
Quality Control in Manufacturing
In quality control, binomial distribution is used to predict the number of defective items in a batch. Manufacturers set acceptance criteria based on binomial probabilities to ensure that the proportion of defective items does not exceed a certain threshold. This helps in managing risks associated with product quality.
A/B Testing in Marketing
A/B testing involves comparing two versions of a marketing strategy to determine which one performs better. Binomial distribution helps in evaluating the success of these strategies by analyzing binary outcomes such as click-through rates or conversion rates. This aids in managing marketing risks by optimizing campaigns based on data-driven insights.
Monte Carlo Simulations
Monte Carlo simulations use binomial distribution to model uncertainty and estimate outcomes over numerous trials. By simulating binary events repeatedly, companies can assess various risk scenarios and make informed decisions under uncertainty.
Case Studies and Examples
Several real-world examples illustrate the successful application of binomial distribution:
-
Banking: A major bank used binomial distribution to develop a credit scoring model that significantly reduced default rates by accurately predicting borrower creditworthiness.
-
Insurance: An insurance company applied binomial distribution in actuarial science to predict claim frequencies and adjust premiums accordingly.
-
Investment Firms: A hedge fund utilized the binomial options pricing model to accurately price options and implement effective hedging strategies.
These case studies highlight how binomial distribution has been instrumental in improving decision-making processes across various financial sectors.
Nguồn: https://linegraph.boats
Danh mục: Blog